3. d-separation, Graph Transformation
In a different place, we talked about direction-dependent separation, or d-separation. In this notebook, we will explore a different way to compute d-separation based on graph transformation.
3.1. Graph example
Here is a graph taken from this most excellent video tutorial on d-separation exercises. We will be using this graph to show some d-separation queries.
[1]:
import networkx as nx
from collections import namedtuple
Graph = namedtuple('Graph', 'u, d')
def get_edges(s):
edges = s.split('\n')
edges = map(lambda line: line.split('->'), edges)
edges = map(lambda tokens: tuple([t.strip() for t in tokens]), edges)
edges = list(edges)
return edges
def get_directed_graph(edges):
g = nx.DiGraph()
g.add_edges_from(edges)
return g
def get_undirected_graph(edges):
g = nx.Graph()
g.add_edges_from(edges)
return g
def get_graph(s):
e = get_edges(s)
d = get_directed_graph(e)
u = get_undirected_graph(e)
g = Graph(u, d)
return g
[2]:
s = '''
U -> W
V -> W
V -> X
V -> T
W -> Y
X -> Y
T -> Z
'''.strip()
g = get_graph(s)
[3]:
import matplotlib.pyplot as plt
pos = nx.nx_agraph.graphviz_layout(g.d, prog='dot')
fig, ax = plt.subplots(figsize=(5, 5))
nx.draw_networkx_nodes(
G=g.d,
pos=pos,
ax=ax,
node_size=100,
alpha=0.5,
node_color='red'
)
nx.draw_networkx_labels(
G=g.d,
pos=pos,
ax=ax,
font_size=15,
font_color='black',
alpha=1.0
)
nx.draw_networkx_edges(
G=g.d,
pos=pos,
alpha=0.25,
arrowsize=30,
ax=ax
)
fig.tight_layout()
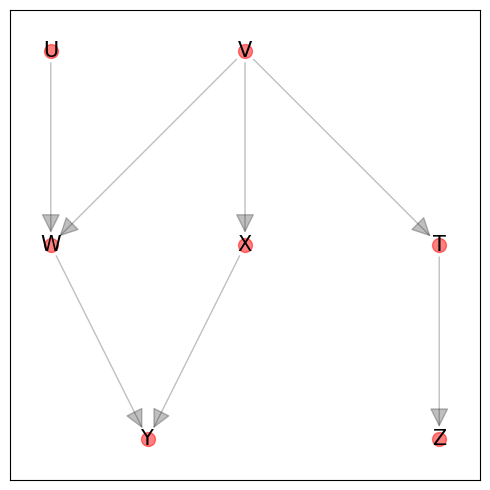
3.2. Algorithm
Here’s the graph transformation algorithm to determine if \(X\) and \(Y\) are d-separated given \(Z\).
Input:
\(G\): DAG
\(X\): start node
\(Y\): end node
\(Z\): evidence nodes
Output: True or False
Create the ancestral graph \(A\) from \(G\) (using the starting nodes in \(X, Y, Z\))
Create the moralized graph \(M\) from \(A\)
Create the dependency graph \(D\) from \(M\) by remove all nodes in \(Z\) and their associated edges
If there is no path between \(X\) and \(Y\) in \(D\), return true, else false
[4]:
import itertools
def get_ancestral_graph(g, x, y, z):
seen = []
nodes = [x, y] + list(z)
while True:
seen = seen + [n for n in nodes if n not in seen]
parents = [list(g.d.predecessors(n)) for n in nodes]
parents = itertools.chain(*parents)
parents = set(parents)
parents = parents - set(seen)
parents = list(parents)
if len(parents) < 1:
break
else:
nodes = parents
a = g.u.copy()
for n in g.u.nodes():
if n not in seen:
a.remove_node(n)
return a
def get_moralized_graph(g, a, x, y, z):
m = a.copy()
for n in a.nodes():
pa_links = itertools.combinations(list(g.d.predecessors(n)), 2)
for pa_1, pa_2 in pa_links:
if pa_1 in m.nodes() and pa_2 in m.nodes() and not m.has_edge(pa_1, pa_2):
m.add_edge(pa_1, pa_2)
return m
def get_dependency_graph(m, evidence=set()):
d = m.copy()
for n in evidence:
d.remove_node(n)
return d
def is_d_separated(g, x, y, evidence=set()):
a = get_ancestral_graph(g, x, y, evidence)
m = get_moralized_graph(g, a, x, y, evidence)
d = get_dependency_graph(m, evidence)
if x not in d.nodes() or y not in d.nodes() or not nx.has_path(d, x, y):
return True
return False
3.3. Examples
Below are some exercise examples to query for d-separation based on the algorithm and code above.
3.3.1. Example 1
[5]:
False == is_d_separated(g, 'V', 'Z')
[5]:
True
3.3.2. Example 2
[6]:
True == is_d_separated(g, 'V', 'Z', {'T'})
[6]:
True
3.3.3. Example 3
[7]:
True == is_d_separated(g, 'U', 'V')
[7]:
True
3.3.4. Example 4
[8]:
False == is_d_separated(g, 'U', 'V', {'W'})
[8]:
True
3.3.5. Example 5
[9]:
True == is_d_separated(g, 'U', 'V', {'X'})
[9]:
True
3.3.6. Example 6
[10]:
False == is_d_separated(g, 'U', 'V', {'Y'})
[10]:
True
3.3.7. Example 7
[11]:
True == is_d_separated(g, 'U', 'V', {'Z'})
[11]:
True
3.3.8. Example 8
[12]:
False == is_d_separated(g, 'W', 'X')
[12]:
True
3.3.9. Example 9
[13]:
True == is_d_separated(g, 'X', 'T', {'V'})
[13]:
True
3.3.10. Example 10
[14]:
False == is_d_separated(g, 'X', 'W', {'U'})
[14]:
True
3.3.11. Example 11
[15]:
False == is_d_separated(g, 'Y', 'Z')
[15]:
True
3.3.12. Example 12
[16]:
True == is_d_separated(g, 'Y', 'Z', 'T')
[16]:
True
3.3.13. Example 13 **
[17]:
False == is_d_separated(g, 'Y', 'Z', {'X'})
[17]:
True
3.3.14. Example 14
[18]:
True == is_d_separated(g, 'Y', 'Z', {'V'})
[18]:
True
3.3.15. Example 15
[19]:
True == is_d_separated(g, 'W', 'Z', {'V'})
[19]:
True
3.3.16. Example 16
[20]:
True == is_d_separated(g, 'U', 'Z')
[20]:
True
3.3.17. Example 17
[21]:
False == is_d_separated(g, 'U', 'Z', {'Y'})
[21]:
True
3.3.18. Additional examples
[22]:
False == is_d_separated(g, 'U', 'W')
[22]:
True
[23]:
True == is_d_separated(g, 'U', 'W', {'U'})
[23]:
True
[24]:
True == is_d_separated(g, 'U', 'W', {'W'})
[24]:
True
[ ]: