3. Optimizing Marginal Revenue from the Demand Curve
The demand curve is usually defined as a plot of demand/quantity (y-axis), \(Q\), against the price (x-axis), \(P\). For nearly all products, as \(P\) goes up then \(Q\) comes down. We can model the demand curve (linearly or non-linearly) to figure out how \(P\) and \(Q\) are related, and use this relationship to find the “optimal” price that will maximize marginal revenue. Let’s see how computing the optimal price from a demand curve works.
3.1. Simulated data
Let’s simulate some data for \(P\) and \(Q\).
\(P \sim \mathcal{N}(40, 20)\)
\(Q \sim \mathcal{N}(400 - 5.0 P, 1)\)
[1]:
import pandas as pd
import numpy as np
np.random.seed(37)
N = 100
P = np.random.normal(40, 20, N) # np.arange(0, 81, 1)
Q = np.random.normal(400 + (-5 * P), 1, P.shape[0]) + np.random.normal(10, 20, N)
df = pd.DataFrame({'p': P, 'q': Q}) \
.sort_values(['p']) \
.query('p >= 0') \
.reset_index(drop=True)
df.shape
[1]:
(97, 2)
As you can see below, the demand curve is not smooth and often times, this seesaw curve is the empirical demand curve of a lot of products. We need to smooth it out.
[2]:
import matplotlib.pyplot as plt
f, a = plt.subplots(figsize=(7, 3))
df.set_index(['p'])['q'] \
.plot(
kind='line',
ax=a,
title='Demand curve',
ylabel='q'
)
f.tight_layout()
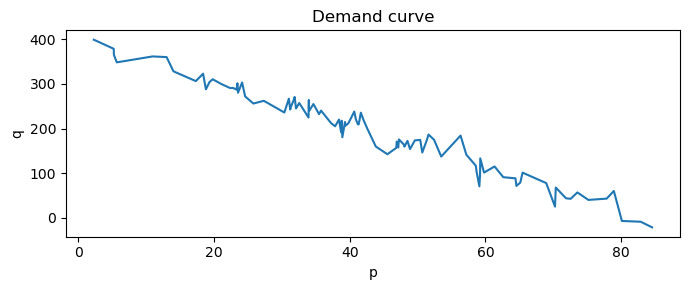
3.2. Modeling
We can model the demand curve with linear regression. The linear regression model of the demand curve is a straight line (it smooths out the empirical demand curve).
\(Q \sim P\)
[3]:
from sklearn.linear_model import LinearRegression
X = df[['p']]
y = df['q']
qp_model = LinearRegression()
qp_model.fit(X, y)
qp_model.intercept_, qp_model.coef_
[3]:
(402.0611487648074, array([-4.83565712]))
[4]:
pred_df = df.assign(q_pred=qp_model.predict(X))
f, a = plt.subplots(figsize=(7, 3))
pred_df \
.rename(columns={'q': 'q_true'}) \
.set_index(['p']) \
.plot(
kind='line',
ax=a,
title='Empirical and modeled demand curves',
ylabel='q'
)
f.tight_layout()
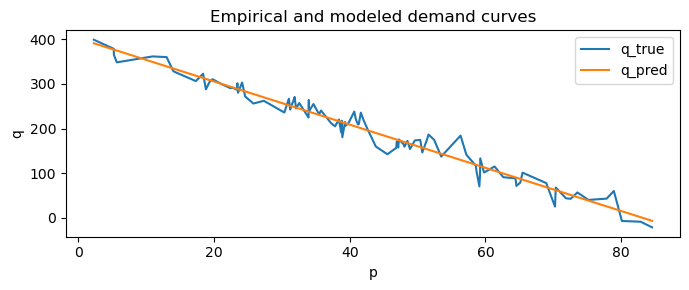
3.3. Marginal revenue optimization
From the linear regression model of the demand curve, we have the following relationships from the model.
\(Q = 401.96 - 4.8 P\)
\(P = 83.74 - 0.21 Q\)
We know the following about total revenue, \(R\).
\(R = P Q\)
\(R = \left(83.74 - 0.21 Q \right) Q\)
\(R = 83.74Q - 0.21 Q^2\)
The marginal revenue is \(R' = \dfrac{\mathrm{d} R}{\mathrm{d} Q}\).
\(R' = \dfrac{\mathrm{d} R}{\mathrm{d} Q} = 83.74 - 0.42 Q\)
The marginal cost, \(C'\), of producing a unit is (given as) 15.0. The optimal price can be found when we set \(R' = C'\) and solve for \(Q\) and plug back in \(Q\) to get \(P\).
\(R' = C'\)
\(83.74 - 0.42 Q = 15.0\)
\(-0.42 Q = -68.74\)
\(Q = 163.67\)
Since we know \(P = 83.74 - 0.21 Q\), we plug \(Q\) in to get the price.
\(P = 83.74 - 0.21 Q\)
\(P = 83.74 - 0.21 (163.67)\)
\(P = 49.37\)
Let’s compute \(R = PQ\).
\(R = PQ\)
\(R = 49.37 \times 163.67\)
\(R = 8,080.39\)
Let’s compute \(R'\).
\(R' = 83.74 - 0.42 Q\)
\(R' = 83.74 - 0.42 (163.67)\)
\(R' = 15.00\)
Let’s define the profit, \(T\), as follows, and plug in the values.
\(T = PQ - C'Q\)
\(T = (49.37 \times 163.67) - (15 \times 163.67)\)
\(T = 5,625.34\)
[5]:
def get_pq(b_0, b_1):
return lambda q: (-b_0 / b_1) + (1 / b_1) * q
def get_qp(b_0, b_1):
return lambda p: b_0 + (b_1 * p)
def get_mr(b_0, b_1):
return lambda q: (-b_0 / b_1) + (2 * (1 / b_1) * q)
def get_qo(b_0, b_1):
z_0 = (-b_0 / b_1)
z_1 = 2 * (1 / b_1)
return lambda mc: (mc - z_0) / z_1
def get_funcs(b_0, b_1):
pq = get_pq(b_0, b_1)
qp = get_qp(b_0, b_1)
mr = get_mr(b_0, b_1)
qo = get_qo(b_0, b_1)
r = lambda p, q: p * q
t = lambda p, q, mc: (p * q) - (mc * q)
return pq, qp, mr, qo, r, t
def get_opt(f, mc):
pq_f, qp_f, mr_f, qo_f, r_f, t_f = f
q_opt = qo_f(mc)
p_opt = pq_f(q_opt)
mr_opt = mr_f(q_opt)
r_opt = r_f(p_opt, q_opt)
t_opt = t_f(p_opt, q_opt, mc)
return pd.Series({
'mc': mc,
'q_opt': q_opt,
'p_opt': p_opt,
'mr_opt': mr_opt,
'r_opt': r_opt,
't_opt': t_opt
})
f = get_funcs(qp_model.intercept_, qp_model.coef_[0])
For this product, the optimal price is 49.08 which will lead to
164.72 units sold (quantity),
8,084.68 USD in revenue, and
5,613,77 USD in profit.
[6]:
get_opt(f, 15)
[6]:
mc 15.000000
q_opt 164.763146
p_opt 49.072545
mr_opt 15.000000
r_opt 8085.346941
t_opt 5613.899751
dtype: float64
The plot below shows the marginal revenue mr
, total revenue tr
and profit pr
at each price point p
. The vertical red dotted line marks the optimal price and it intersects with the profit curve an its highest point.
[7]:
fig, ax = plt.subplots(figsize=(7, 3))
pred_df \
.assign(
mr=lambda d: f[2](d['q_pred']),
tr=lambda d: f[4](d['p'], d['q_pred']),
pr=lambda d: f[5](d['p'], d['q_pred'], 15)
) \
.set_index(['p'])[['mr', 'tr', 'pr']] \
.plot(kind='line', ylabel='USD', ax=ax)
ax.axvline(x=get_opt(f, 15).p_opt, color='r', alpha=0.5, linestyle='dotted')
ax.legend(bbox_to_anchor=(1.1, 1.05))
fig.tight_layout()
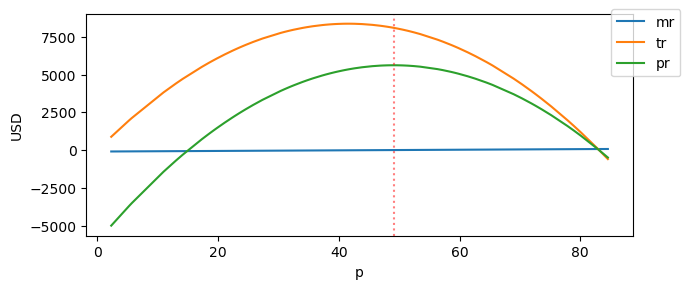