8. K-means, BIC, AIC
8.1. Data
[1]:
import numpy as np
import random
from numpy.random import normal
from collections import namedtuple
Data = namedtuple('Data', 'X y')
random.seed(37)
np.random.seed(37)
def get_data(means, variances, labels, N=1000):
def get_X(sample_means, sample_variances, N):
return np.hstack([normal(m, v, N).reshape(-1, 1) for m, v in zip(sample_means, sample_variances)])
def get_y(label, N):
return np.full(N, label, dtype=np.int)
X = np.vstack([get_X(m, v, N) for m, v in zip(means, variances)])
y = np.hstack([get_y(label, N) for label in labels])
return Data(X, y)
# training
means = [
[5.0, 5.0],
[99.0, 99.0],
[15.0, 20.0]
]
variances = [
[1.0, 1.0],
[1.0, 1.0],
[1.0, 1.0]
]
labels = range(len(means))
X, y = get_data(means=means, variances=variances, labels=labels)
print(f'X shape = {X.shape}, y shape {y.shape}')
X shape = (3000, 2), y shape (3000,)
8.2. BIC, AIC and more
[2]:
from sklearn.cluster import KMeans
from sklearn.metrics import silhouette_score, davies_bouldin_score
from sklearn.metrics import homogeneity_score, completeness_score, v_measure_score
from sklearn.metrics import calinski_harabasz_score
from sklearn.mixture import GaussianMixture
from scipy.stats import multivariate_normal as mvn
def get_km(k, X):
km = KMeans(n_clusters=k, random_state=37)
km.fit(X)
return km
def get_bic_aic(k, X):
gmm = GaussianMixture(n_components=k, init_params='kmeans')
gmm.fit(X)
return gmm.bic(X), gmm.aic(X)
def get_score(k, X, y):
km = get_km(k, X)
y_pred = km.predict(X)
bic, aic = get_bic_aic(k, X)
sil = silhouette_score(X, y_pred)
db = davies_bouldin_score(X, y_pred)
hom = homogeneity_score(y, y_pred)
com = completeness_score(y, y_pred)
vms = v_measure_score(y, y_pred)
cal = calinski_harabasz_score(X, y_pred)
return k, bic, aic, sil, db, hom, com, vms, cal
The meaning of the scores.
BIC
lower is betterAIC
lower is bettersilhouette
higher is betterdavies
lower is betterhomogeneity
higher is bettercompleteness
higher is betterv-measure
higher is bettercalinski
higher is better
[3]:
import pandas as pd
df = pd.DataFrame([get_score(k, X, y) for k in range(2, 11)],
columns=['k', 'BIC', 'AIC', 'silhouette',
'davies', 'homogeneity',
'completeness', 'vmeasure', 'calinski'])
df
[3]:
k | BIC | AIC | silhouette | davies | homogeneity | completeness | vmeasure | calinski | |
---|---|---|---|---|---|---|---|---|---|
0 | 2 | 29713.687099 | 29647.617056 | 0.941677 | 0.082989 | 0.57938 | 1.000000 | 0.733680 | 1.827224e+05 |
1 | 3 | 23709.628278 | 23607.520029 | 0.929479 | 0.099672 | 1.00000 | 1.000000 | 1.000000 | 2.623227e+06 |
2 | 4 | 23764.812594 | 23626.666140 | 0.731644 | 0.660094 | 1.00000 | 0.828906 | 0.906450 | 1.963947e+06 |
3 | 5 | 23812.883924 | 23638.699264 | 0.504188 | 1.032971 | 1.00000 | 0.703982 | 0.826279 | 1.672838e+06 |
4 | 6 | 23856.161363 | 23645.938498 | 0.306449 | 1.264110 | 1.00000 | 0.613262 | 0.760275 | 1.541983e+06 |
5 | 7 | 23898.831307 | 23652.570237 | 0.313627 | 1.128982 | 1.00000 | 0.573512 | 0.728958 | 1.437240e+06 |
6 | 8 | 23941.542123 | 23659.242848 | 0.324070 | 1.015535 | 1.00000 | 0.534216 | 0.696402 | 1.407921e+06 |
7 | 9 | 23986.361374 | 23668.023893 | 0.329671 | 0.952718 | 1.00000 | 0.502211 | 0.668629 | 1.422252e+06 |
8 | 10 | 24035.079248 | 23680.703562 | 0.326965 | 0.955671 | 1.00000 | 0.480658 | 0.649249 | 1.366897e+06 |
8.3. Visualize
[4]:
%matplotlib inline
import matplotlib.pyplot as plt
from sklearn.preprocessing import MinMaxScaler
plt.style.use('ggplot')
def plot_compare(df, y1, y2, x, fig, ax1):
ax1.plot(df[x], df[y1], color='tab:red')
ax1.set_title(f'{y1} and {y2}')
ax1.set_xlabel(x)
ax1.set_ylabel(y1, color='tab:red')
ax1.tick_params(axis='y', labelcolor='tab:red')
ax2 = ax1.twinx()
ax2.plot(df[x], df[y2], color='tab:blue')
ax2.set_ylabel(y2, color='tab:blue')
ax2.tick_params(axis='y', labelcolor='tab:blue')
def plot_contrast(df, y1, y2, x, fig, ax):
a = np.array(df[y1])
b = np.array(df[y2])
r_min, r_max = df[y1].min(), df[y1].max()
scaler = MinMaxScaler(feature_range=(r_min, r_max))
b = scaler.fit_transform(b.reshape(-1, 1))[:,0]
diff = np.abs(a - b)
ax.plot(df[x], diff)
ax.set_title('Scaled Absolute Difference')
ax.set_xlabel(x)
ax.set_ylabel('absolute difference')
def plot_result(df, y1, y2, x):
fig, axes = plt.subplots(1, 2, figsize=(10, 3))
plot_compare(df, y1, y2, x, fig, axes[0])
plot_contrast(df, y1, y2, x, fig, axes[1])
plt.tight_layout()
8.3.1. BIC vs silhouette
[5]:
plot_result(df, 'BIC', 'silhouette', 'k')
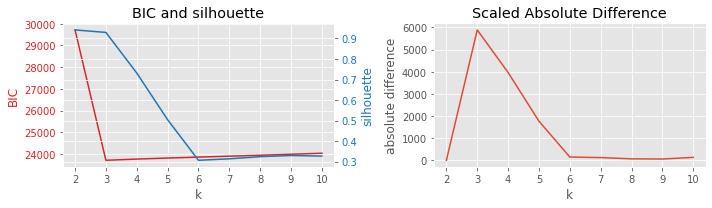
8.3.2. BIC vs Davies-Bouldin
[6]:
plot_result(df, 'BIC', 'davies', 'k')
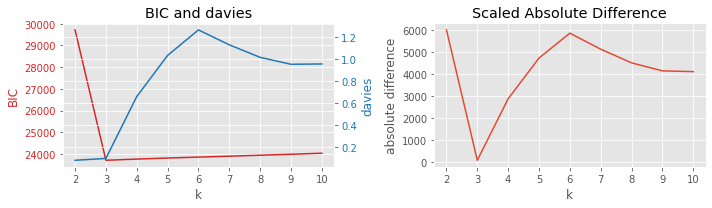
8.3.3. BIC vs homogeneity
[7]:
plot_result(df, 'BIC', 'homogeneity', 'k')
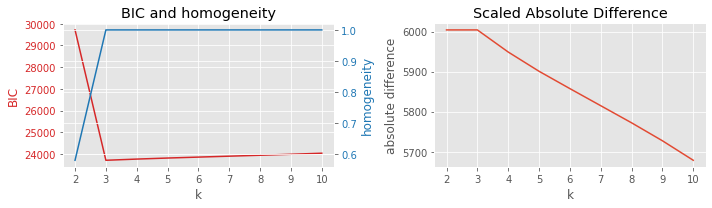
8.3.4. BIC vs completeness
[8]:
plot_result(df, 'BIC', 'completeness', 'k')
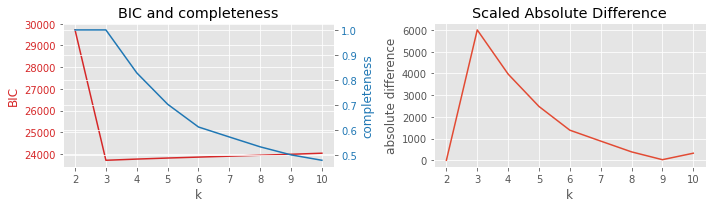
8.3.5. BIC vs Calinski-Harabasz
[9]:
plot_result(df, 'BIC', 'calinski', 'k')
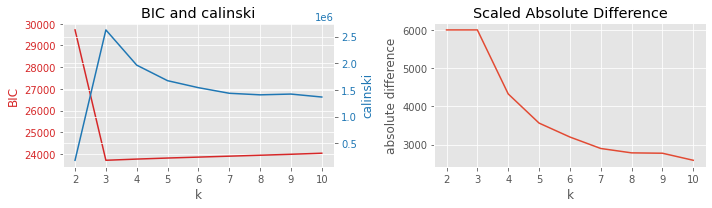
8.3.6. BIC vs v-measure
[10]:
plot_result(df, 'BIC', 'vmeasure', 'k')
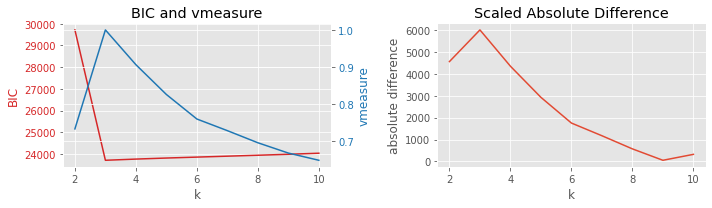